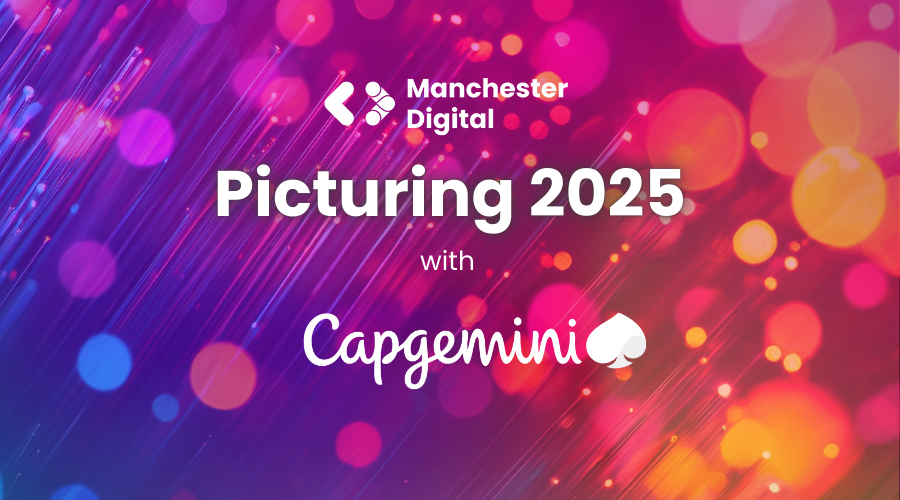
As we approach 2025, Manchester Digital is excited to share Picturing 2025 - a series of essays from our members offering insights into the tech trends and challenges ahead. Below, Capgemini discuss how GenAI is shaping our digital future.
Generative AI (GenAI) has firmly embedded itself into our daily lives, with 96% of executives worldwide highlighting it as a hot topic in their boardrooms (Capgemini Research). It's being hailed as the AI industry's equivalent of the iPhone moment—a transformative leap that's reshaping how organisations operate. Companies are already using GenAI for hyper-personalised marketing or to turn those previously unloved chatbots into sophisticated assistants, perfectly tuned to mirror a brand's unique tone of voice.
But while businesses are busy seizing the opportunities GenAI offers, the next big thing is already making waves: enter AI Agents. Real-world use cases are finally emerging and it is about to get very exciting whether they can live up to the hype or not.
So, what exactly are AI agents, and how do they work? Let’s delve into their concept and explore the possibilities they bring.
Currently, most people use a Large Language Model (LLM) to perform a specific task: write an article, generate code, summarise text etc. Thousands of models exist, such as ChatGPT, some specialized for specific tasks and others more general. But in an agentic workflow you have multiple agents (=LLMs) forming an AI-driven system that is capable of understanding instructions, making decisions, and performing actions. This is no possible due to the advanced reasoning capabilities of modern language models.
At the core of this setup is a planner agent, which acts as the orchestrator, crafting a step-by-step strategy based on user input. For instance, if the task is to schedule a vehicle, the planner would create a plan like this: First retrieve customer ID, then check vehicle status, next book the delivery and lastly notify the customer by email.
The planner then delegates these tasks to specialized agents, each tailored for a specific function. A database retrieval agent might handle queries, while a customer communication agent drafts and sends emails. Additional layers, like a quality assurance agent, can verify outputs to ensure accuracy.
These systems operate iteratively, with agents exchanging updates and refining their actions until the goal is achieved. Their interactions often follow a structured sequence of reasoning (THOUGHT), task execution (TASK), and action refinement (ACTION), adapting dynamically if challenges arise.
Observing these AI models "communicate" and problem-solve in real-time provides a glimpse into the power and potential of agentic AI systems, showcasing how they can work together seamlessly to execute complex, multi-step workflows. If one agent encounters an error, the other agents dynamically try to solve the problem between them until they achieve their goal, by retrying queries or rewriting code.
Where might these systems find practical application? A relatable example is scheduling a meeting among five participants—a task that often consumes significant time as individuals struggle to align their calendars, only to face repeated rescheduling. An agentic system could streamline this process: a planner agent could define the task, a calendar agent could retrieve participants' availability, and a communication agent could confirm suitable times with each attendee. By iterating dynamically until an optimal slot is identified, such a system could save countless hours of employee productivity each year.
2025 is THE year where AI agents are deployed at scale and as we navigate their potential, key questions arise: How much autonomy do we want to give agents? What frameworks will govern these systems? These are all questions the industry is actively exploring and will continue to address in 2025 as we introduce agents into enterprise environments.
We’re just at the beginning of this journey but either way, the pace of technological change shows no signs of slowing.
By Dr. Katharina Reusch – Senior Tech Lead for GenAI @ Capgemini