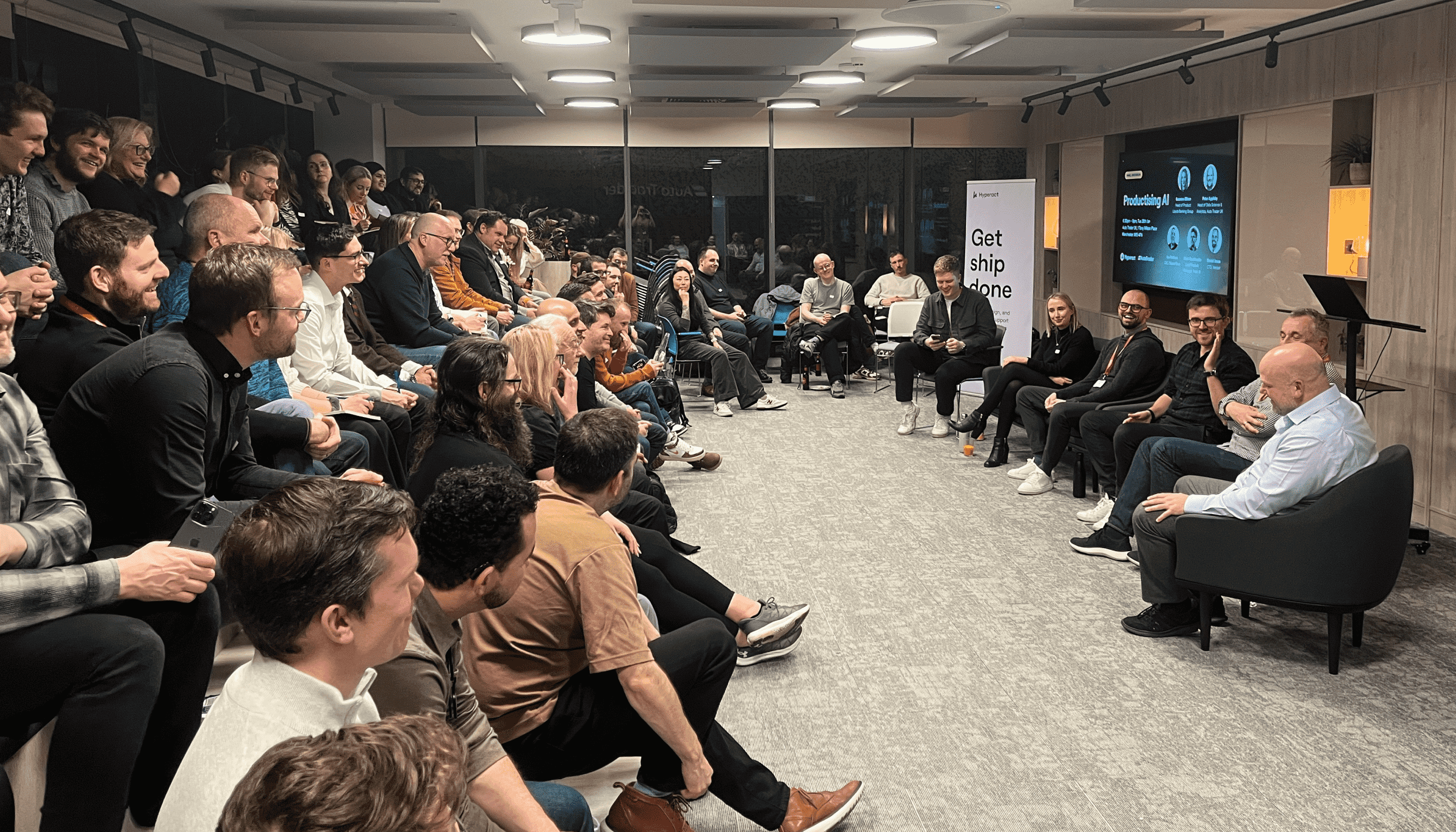
We recently hosted our first panel discussion in Manchester, "Productising AI: From hype to value," at Auto Trader UK. The event brought together over 100 product and technology leaders in the region to discuss the challenges and opportunities of bringing AI products to market.
The realities of productising AI
The panel:
- Mark Douthwaite, Lead Product Manager, Peak AI
- Ian Pattison, CEO, Neural River
- Suzanne Ellison, Head of Product, Lloyds Banking Group
- Daniel Jones, CTO, Versori
- Peter Appleby, Head of Data Science & Analytics, Auto Trader UK
Each panelist shared their experiences and insights into the realities of productising AI.
- What they’ve learnt bringing AI products to market
- Augmenting existing products and services with AI
- Where AI products are heading
The discussion covered various aspects, including defining AI products, lessons learned from taking AI products from concept to launch, balancing customer expectations with real-world value, and addressing ethical, technical, and operational AI risks.
Key takeaways
From a product perspective, building an AI product vs building a more traditional product or service should be treated the same. Make sure it is genuinely useful and valuable to customers:
- Don't get caught up in the hype.
Stick to solid product principles and continue to prioritise commercial viability, data integrity, and customer value.
- Not every challenge needs AI.
Determine whether AI is necessary to solve a particular problem or if a simpler, more effective approach can be taken. Don't force a square peg into a round hole. Challenges assumed to be solvable by AI can likely be solved much more cost-effectively and earlier in the data lifecycle.
- Time and risk management are critical.
Building an AI product requires careful testing and assurance around bias, trust, and reliability. You must allow extra time for testing. Particularly given that traditional software testing methods might not be adequate for testing novel solutions that are often non-deterministic.
As is the case for most Large Language Models (LLMs). Extra time gives your team the buffer it needs to catch unforeseen issues, and the time to research emerging techniques. Catching issues early, before they snowball.
- Education is key to building trust.
Educating users and customers about what AI can, and cannot do, is crucial for building trust and setting realistic expectations. You can’t assume everyone understands AI. If you are looking to build an AI product, then take the time to clearly explain its capabilities and limitations upfront.
In doing so, you help stakeholders have an accurate perspective from the start. This is especially important to communicate to your customers too.
- Ethical concerns must be addressed.
Openness, ethical AI deployment, and educating users on its capabilities are crucial for building trust and ensuring AI benefits the common good. It's not just about developing AI products. It's about making it work for everyone. Looking ahead, we wouldn’t be surprised to see accreditation introduced for developers to ensure robust safeguards protect users.
Expert insights from the panel
Suzanne Ellison: AI Products are just like any other – they need to add customer and business value. Start with the problem and use the tech to solve it! Your AI is only as good as the data, rigorous testing is important as well as ongoing monitoring. You need to manage the positioning of AI solutions carefully so that clear expectations are managed and you build trust with users of your product.
Ian Pattison: Don't assume people always know what AI can do. Those making key decisions usually have their heads down and it's up to experts to help guide. Often the solution you need isn't AI. It's a much simpler solution.
Mark Douthwaite: There's a distinction between AI-augmented products and AI-native products. Mark emphasises the importance of understanding the right use cases for each. Peak.AI are proactive in educating their customers through workshops.
Peter Appleby: Auto Trader UK's approach to applying AI to solve specific parts of a challenge is ensuring AI plays to its strengths without becoming the entire solution. Their focus is on areas where AI can really make a difference to users. In Auto Trader's case this is helping car sellers select which features are the most important to highlight to potential car buyers.
Daniel Jones: Has been through the challenges of AI evolving at breakneck speed and have taken extra measures to balance speed with stability. You can't afford to get left behind, but you also can't afford to rush into things.
The audience raised thought-provoking questions, including:
- How can we test AI at scale and ensure it's trustworthy?
- What guardrails and control mechanisms are needed to maintain standards and prevent misuse of AI?
- How can we reuse capabilities across the business and minimize risk and impact?
Thanks to all those who attended, the panelists and hosts, Auto Trader UK.
The "Productising AI: From Hype to Value" event was an insightful and engaging discussion on the realities of bringing AI products to market. Our panel's experiences and insights provided valuable lessons for professionals looking to navigate the complex world of AI product development.
We're grateful to our panelists, attendees, and Auto Trader UK for making this event possible.
What's next?
If you're interested in staying updated on future Hyperact events and insights, follow us on LinkedIn. What do you think are the most significant challenges in productising AI? Share your thoughts here and let's continue the conversation!