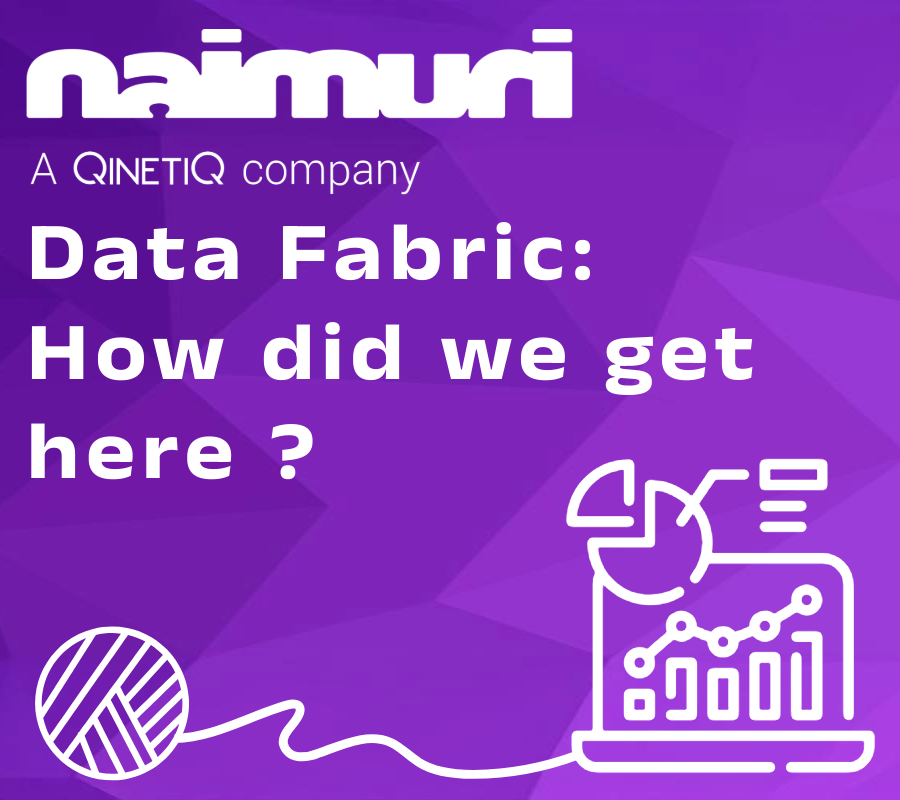
Written by Rose Carney.
As we enter 2025, organisations that manage, process, generate, and derive value from data are facing an increasingly complex landscape. With the rapid rise of AI, data governance and utilisation have never been more challenging or more critical.
It's no surprise, then, that as paradigm-shifting data-driven technologies continue to emerge, businesses are reassessing their existing data architectures. Many are asking: Can we do more with our data? How can we innovate responsibly while ensuring compliance and maintaining ethical standards in our data practices?
Data Fabric often comes up as a potential solution to these questions.
The concept of a "Data Fabric" isn't entirely new. While the idea has been around for several years, it has gained significant traction recently due to its relevance in addressing modern data landscape challenges. But what exactly is a Data Fabric, and why is it essential for tech organisations to understand its potential value when designing data architectures and strategies?
What is Data Fabric?
Data Fabric is often described as a set of guiding principles designed to shape a data architecture focused on creating a unified data layer across your organisation. It represents a natural progression from earlier architectures, addressing their limitations while building on their strengths:
- Data Warehouse: A centralised repository for data with rigid structures, offering a single source of structured truth. However, this approach often sacrifices flexibility, making it difficult to adapt to changing business needs.
- Data Lake(house): Evolved from the Data Warehouse by retaining a centralised repository but removing rigid data structures. This allows data to exist in its raw form, preserving value but creating significant overhead—especially in governance and management.
- Data Mesh: Acknowledges the shortcomings of centralised strategies, particularly the creation of silos between data producers and consumers. While Data Mesh decentralises data ownership, it introduces new challenges, such as ensuring consistent governance, interoperability, and scalable infrastructure.
Data Fabric builds on these foundations, offering a more flexible solution to unify data while addressing the complexities of governance, accessibility, and innovation.
No One-Size-Fits-All Approach
Truthfully, there’s no single set of data architecture principles that can meet the needs of every organisation. In many cases, a legacy Data Warehouse may still deliver critical business value, making it impractical or too costly to replace. At the same time, a department or team within the same organisation might have fully embraced decentralisation, adopting domain-specific data teams in line with Data Mesh principles.
Most likely, there will also be repositories of data with varying structures, accessed by multiple functions across the organisation. These teams might apply their own processes, tools, or interpretations, creating inconsistencies and further complicating governance. While there is often some effort to consolidate these diverse strategies into a cohesive long-term approach, organisations are increasingly turning to Data Fabric as a practical solution for the immediate challenges. By unifying different methods of storing and serving data, Data Fabric provides a framework to bridge these fragmented systems and enable seamless, scalable data management.
Key Concepts of Data Fabric
So, what are the key concepts behind Data Fabric? Let's explore the components:
1 - Unified Data Access and Integration
As previously mentioned, this is a core feature of Data Fabric. It eliminates data silos and provides a consistent view of data, enabling organisations to leverage all their data seamlessly, regardless of location or format.
2 - Metadata and Cataloguing
Data Fabric heavily relies on metadata to provide context, enable automation, and ensure proper governance. Cataloging your data is crucial when it comes to both exploitation and governance. Today, there are a variety of open-source, cloud-native, or proprietary options for businesses to explore, each offering varying features such as data lineage and versioning.
3 - Virtualisation
The concept of Data Fabric includes integrating data sources into a unified layer, but that doesn’t necessarily mean lifting and shifting data. Virtualisation allows data to be accessed without requiring physical replication or movement. This reduces latency and storage costs while ensuring that data remains accessible and actionable in real time.
4 - Automation and Intelligence
Data Fabric embraces the use of Artificial Intelligence in data management. Tasks such as integration, quality checks, and governance can be automated, improving efficiency and ensuring consistent data quality across the organisation.
These key components are fundamental to Data Fabric and need to be fully considered by organisations looking to adopt it. It is not a prescriptive set of tools and technologies but rather a set of principles that inform leadership decisions about where to invest, helping organisations avoid the pitfalls of past architectures.