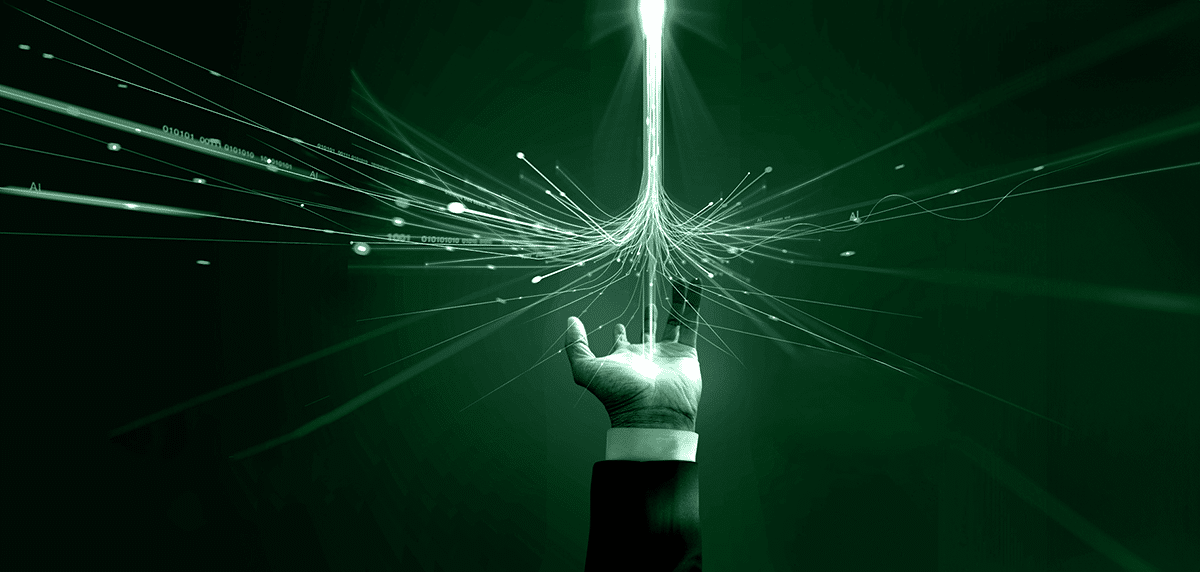
Artificial Intelligence (AI) promises to bring enormous benefits to society while also transforming business and industry by creating much faster and smarter ways of completing a myriad of tasks.
At the same time, AI applications will create increasing demand for data center space and consume considerable power. The latest NVIDIA GB200 rack cabinet configuration (aka Grace Blackwell), for example, is >120kW. And according to a recent study a Generative AI system could use around 33 times more energy than machines running task-specific software. Furthermore, the UK National Grid has said that data center power demand in the UK will rise six-fold in just 10 years, fueled largely by the rise of AI.
If any further proof was required for the demand for power, at nLighten we’ve seen requirements for 130kW racks and have already deployed racks at 42kW. To put this into perspective, the power consumption of an IT rack was once 2 to 3 kW, while a typical home may only consume about 2.5 kW.
AI demands massive computing resources and generates substantial data.
There are various types of AI: Machine Learning (ML) and its advanced counterparts Deep Learning (DL) and Generative Artificial Intelligence (GenAI). ML has been around for a while and is increasingly deployed not only the academic, scientific, healthcare and government research sectors, but also across industry and commercial enterprises.
However, DL extends beyond ML by automating the learning process after the initial training phase, enabling it to learn and improve without ongoing human intervention GenAI takes it further by generating human-like responses often using massive data-sets to mimic creativity. ChatGPT is a typical example. It leverages Large Language Models (LLMs) to process vast amounts of data and generate human-like text. LLMs are a subset of AI, specifically designed to understand, generate, and manipulate natural language at an impressive scale, making them pivotal in applications like chatbots, content creation, and advanced decision-making. The increasing interest in ML and GenAI will only serve to accelerate the volume of big data that needs to be processed and stored. It is data and lots of it that makes these tick.
All three AI types require Learning and Inference, the former by using training models on large datasets (Big Data) and the latter for applying the trained models to new data. This is how they make predictions or take specific actions.
We’re still very much at the beginning of the AI journey. However, as society continues to generate more and more data thorough our use of technology and its applications, we know AI offers huge potential to drive innovation in many sectors from smart cities, the IoT and autonomous vehicles to healthcare, scientific research and much more.
Taking a hybrid approach to AI
Given the growing demands of AI, a hybrid data center model is emerging as the most effective solution, balancing the strengths of both centralised and edge data centers.
Centralised Data Centers for AI Learning
The training phase of AI, which involves building models based on large datasets, requires vast computational power. Centralised data centers—often located in non-metropolitan areas where power and land are cheaper—are ideal for this resource-heavy process. These large facilities can house the high-performance computing (HPC) infrastructure needed for training AI models.
Edge Data Centers for AI Inference
The inference phase of AI, where models apply their training to make real-time decisions, is better suited for edge data centers. These smaller, distributed data centers are closer to end users and devices, enabling low-latency real-time processing for applications like autonomous vehicles, medical diagnostics, and smart devices. By decentralising inference tasks, edge data centers therefore reduce reliance on central hubs, improving performance and efficiency. In the case of autonomous vehicles for example a millisecond can be the difference between harmony and chaos. The same applies to other applications such as AR and gaming.
Edge data centers can also mitigate rising power usage challenges by not relying totally on one large data center where electricity costs might already be high. Some of the power load can be shared across several energy efficient edge data centers in regions where power may be cheaper. There is also the benefit of disparate sustainable energy generation sites that would be out of reach of centralised hubs
Furthermore, partnering with edge data center operators such as nLighten who are working with local energy providers to support grid stabilization and local heat re-use initiatives can further support sustainability and carbon free credentials. This also helps alleviate demand on land, electricity and water in cities and regions of Europe that are already under significant stress – the so-called FLAP-D markets for example.
Good to know:
Latency Reduction: Edge data centers are designed to be located closer to the users and devices that generate and consume data. This proximity significantly reduces the distance data must travel, which significantly lowers latency and provides faster, real-time responses. This is particularly important for Inference in AI applications due to the requirement for processing specific data and making decisions in near real-time.
Bandwidth Efficiency: Transferring data between devices and a centralised data center can consume substantial network bandwidth, potentially leading to network congestion and increased costs. By processing data locally in an edge data center, AI applications can use bandwidth more efficiently and reduce the volume of data that must be sent over the network.
Security & Privacy: Some AI applications handle sensitive or private data that must be protected. Processing this data locally in an edge data center can reduce the risk of data breaches by minimising the amount of data sent over the long-distance network. It also allows for better compliance with data sovereignty laws which mandate certain types of data to be stored and processed within specific geographical locations.
Reliability and Redundancy: Edge data centers can continue to operate even if the network connection to the centralised data center is lost. This can be vital for AI applications where constant uptime is necessary, such as healthcare or industrial automation.
Scalability: Edge data centers provide a way to increase capacity as needed without significantly impacting the centralised infrastructure. This is useful in scenarios where the amount of data being generated is growing, as is often the case with AI applications.
In summary, as demand for AI continues to grow, pursuing an overly centralised IT deployment approach is likely to bring latency-related performance constraints – especially when it comes to Inference. The utilization of edge data centers to complement centralised facilities can therefore offer a more balanced, cost-efficient, and optimized solution.
Can you afford to risk your company’s future AI investment?
Find out how nLighten edge data centers can accommodate your AI requirements and help accelerate your business to the next level www.nLighten.eu