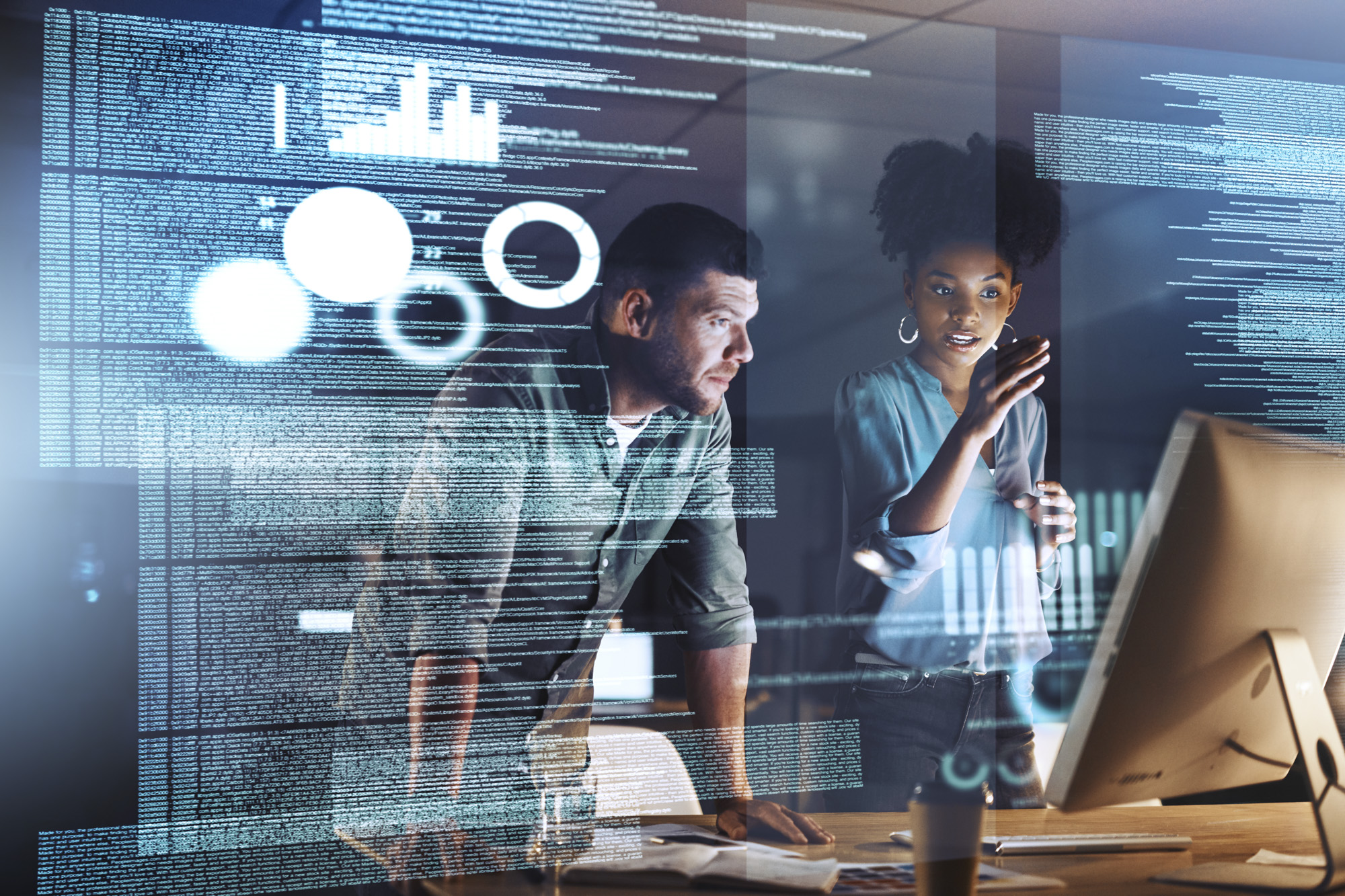
Speaking to a few of the Data Scientists at Zühlke, one of the common themes is that they find it quite difficult to come up with a single definition for what they do. “It’s a pretty exploratory role,” says Simon Debski. “When we sit down with a problem, we don’t know what the answer will be, so as Data Scientists we make a hypothesis, test, assess and repeat the cycle until we’ve solved it. It’s pretty fun.”
Wondering what that looks like in practice? In this article we spoke with Simon, Daphne and Andriy from the Zühlke team to find out more – and why Data Science goes way beyond machine learning and AI.
.
.
Szymon Debski Daphne Michalsen Tsallis Andriy Rekalo
Senior Data Scientist Senior Data Scientist Lead Data ConsultantWhat do Data Scientists do?
Data Science is a broad field that in essence refers to the process of extracting meaning from data. Data Scientists take noisy, messy information and make use of different models and algorithms to provide usable insights. But in reality, this only covers part of their role. Much of the time, they don’t get given a clear brief, and so they need to define their own. According to Andriy Rekalo, one of the Data Scientists at Zühlke, “the most important thing we need to answer isn’t ‘how’ but ‘what’ – what problem should we solve in the first place, what value is in the data and how can I unlock it?”
What do people get wrong about the role of a Data Scientist?
Many people confuse Data Science with Data Engineering, for a start. They are distinct but complementary roles. A Data Engineer is responsible for initially collecting and structuring the data in such a way that it is usable, while a Data Scientist runs analyses, experiments and tests on this data so it can be interpreted. Should one of the models designed by the Data Scientists then be productionised, it is fed back to the Data Engineers for them to take forward. The two work closely together, but they each handle specific parts of a project process.
One thing these three Zühlke Data Scientists can also agree on is that the general public doesn’t really understand what the job entails. “People often hear about buzzword tools and come to you asking to use them – for example, they think that Data Scientists do modelling most of the time,” says Simon.
That’s a sentiment Andriy echoes. “It’s portrayed as being one of the trendiest jobs of the 21st Century, but it’s very different in reality,” he explains. When asked why he says this, he points to all the hard work you have to do before you can get to the ‘cool’ stuff. “Much of your time is spent trying to understand issues with the data and then prepare it for analysis and modelling, and only then can you do things with it,” he says. Having said that, Andriy also highlights the rewarding nature of the role – namely the impact you make with your work in Data Science. “When you see a model deployed you can calculate the tangible value it brings – you can quantify it and give it a number,” he says.
How do you become a Data Scientist?
It’s no surprise given the name, but Data Scientists do spend a lot of time working with data, so it helps to understand how it works. “I’d say you should start by building up a good foundation in statistics, and getting your head around how to handle data is also helpful – especially when there’s a lot of it,” says Daphne Tsallis. Once you’ve got this mastered, you’ll need to add some technical skills too. “You’ll work with data to do tasks like data cleaning, data visualisation and data analysis, so you have to be comfortable with coding – a knowledge of Python or R is useful there,” says Simon.
All of the team members recommend getting some hands-on experience to make sure this is a career that’s actually right for you. Simon suggests signing up for projects and competitions on sites like Kaggle, and then putting a portfolio together before approaching potential employers. This also allows you to follow Andriy’s guidance of finding an aspect of Data Science you really enjoy and developing that further. “My advice would be to resist the pull to focus on the most fashionable technology right now and find something you’re genuinely interested in,” he says.
Why become a Data Scientist?
All three team members get something different out of their roles, and they’re all passionate about what they do. Andriy says it’s the ‘science’ part of Data Science that really appeals to him. “You run experiments and don’t always know where you’re going to end up. There’s way less certainty than a role like traditional software development – but that’s what makes it interesting,” he explains. For Daphne, it’s the scientific part, plus the dynamic nature of the role she appreciates. “I enjoy seeing new signals in the data – it’s exciting to use different theories and experiments to work out why something is happening,” she says.
One key aspect that comes up when talking about Data Science at Zühlke is the community of practice around it. The Data Scientists within the organisation, as well as colleagues interested in the subject, all support each other through formal and informal channels. “It’s all about growing Zühlke’s Data Science capability at the end of the day,” says Simon. He mentions initiatives like building a stock of documentation of past projects to aid with sales and delivery, working together to design new Standard Data Offerings, and doing charitable outreach for non-profits, as just some examples.
A common theme that comes through again and again is the feeling of satisfaction each member of the team gets when all their hard work pays off, and they see the value of their efforts in the real world. This could be as large-scale as helping develop the models behind the NHS Covid-19 Test and Trace App, or something as everyday as automatic claims assessment on an insurance app to make people’s lives just that much easier. Plus, as Simon says, “Even now it still feels like a bit of magic every time you get results.”
In brief...
- Data Scientists work with teams to find out which questions need to be answered using data, and how to best go about doing it.
- It all starts with a solid statistical and mathematical foundation, along with some technical skills to work with the actual data and run experiments.
- Data Science isn’t about any one technology or methodology, but rather finding the right approach to make sense of the data for a given project.
Want to find out more? Head over to our Data Science page and browse open roles here.